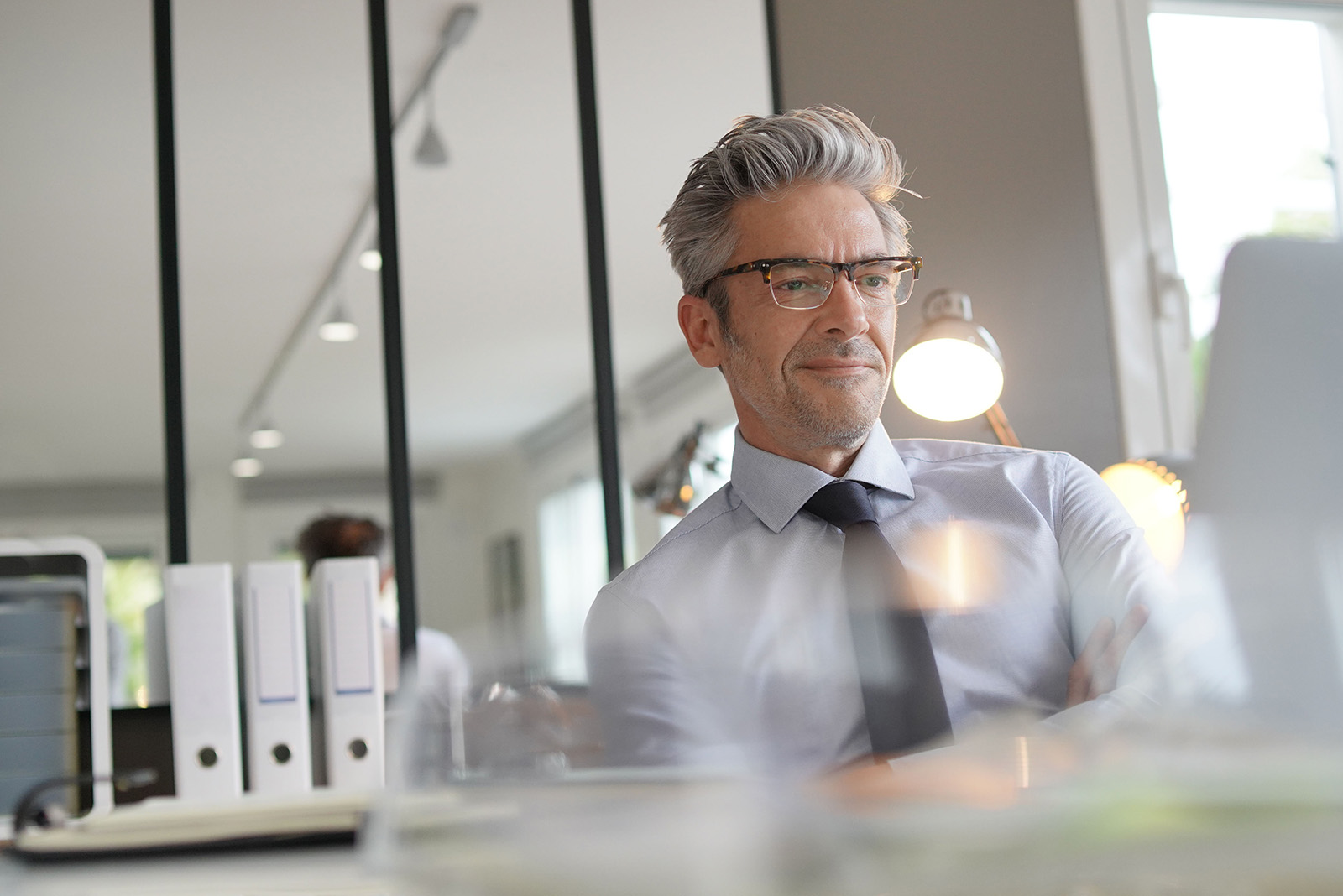
KEITH MANTHEY | Field CTO
Artificial intelligence (AI) transforms industries, drives innovation, and reshapes business operations. However, as AI adoption accelerates, Sysdig reports a nearly 500% increase in AI packages running in workloads over the past year, and so do the security risks. Cybercriminals are increasingly targeting AI systems, taking advantage of vulnerabilities in models, data, and infrastructure. Securing AI workloads requires a proactive and structured approach. Here are five essential steps to ensure that your AI environments remain resilient and secure.
Step 1: Gain Visibility into AI Usage
You can’t protect what you can’t see. The first step to securing AI workloads is understanding where and how AI is deployed across your organization. Many companies unknowingly run AI tools—whether open-source models, third-party APIs, or custom-built systems—without a clear inventory. This lack of visibility creates blind spots that attackers can exploit.
Start by mapping out all AI-related assets: identify the models in use, the frameworks they rely on (like TensorFlow or PyTorch), and the infrastructure hosting them (containers, VMs, or cloud services). Tools like runtime insights can help by providing real-time visibility into active workloads, flagging unexpected behaviors, and pinpointing where AI components reside. Without this foundation, securing your AI ecosystem is like navigating a maze in the dark.
Step 2: Assess and Mitigate Vulnerabilities
AI workloads typically rely on complex software stacks that include various libraries and container images, each of which may harbor vulnerabilities. A single unpatched flaw can jeopardize the entire system. Therefore, it is essential to regularly scan these components for known issues, such as outdated dependencies or misconfigured permissions. This practice is non-negotiable for maintaining security.
Use vulnerability management tools to prioritize risks based on severity and exploitability. For example, a critical flaw in a widely used AI library could allow attackers to inject malicious code or steal sensitive data. Beyond scanning, integrate remediation into your development pipeline. A recent *blog post on Sysdig emphasizes the importance of applying fixes prior to deployment to reduce exposure. This proactive strategy helps ensure that your AI workloads begin with a robust security posture.
Step 3: Secure Data Inputs and Outputs
Data is the lifeblood of AI systems, but it’s also a prime target for attackers. Unprotected data inputs—like training datasets—can be poisoned, skewing model outputs, while exposed outputs can leak confidential information. Recent breaches, like those involving generative AI tools, highlight the stakes: sensitive data extracted via clever prompts can end up in the wrong hands.
Encrypt data at rest and in transit to safeguard your AI workloads. Implement strict access controls, limiting who can feed inputs or view results. Monitor interactions with AI systems to detect anomalies, such as unusual query patterns that might signal an attack. By treating data as a high-value asset, you reduce the risk of compromise.
Step 4: Harden the Runtime Environment
The runtime environment—where AI models execute—is a critical battleground. Attackers often target misconfigured cloud services, weak container settings, or unmonitored processes to gain a foothold. Hardening this environment means locking down configurations and enforcing least-privilege principles.
For instance, ensure containers running AI workloads have minimal permissions and are isolated from unrelated systems. Use runtime security tools to monitor for suspicious activity, like unauthorized network connections or unexpected process launches (think crypto miners or backdoors). In cloud settings, leverage native security features—like AWS IAM roles or Kubernetes network policies—to add layers of defense. A fortified runtime keeps attackers at bay.
Step 5: Monitor and Respond in Real Time
Security isn’t a one-and-done task; it’s an ongoing process. With their dynamic nature, AI workloads require continuous monitoring to catch threats as they emerge. Real-time detection is key—spotting a model behaving oddly or flagging a sudden spike in resource usage.
Deploy monitoring solutions that correlate signals across workloads, cloud logs, and user activity. Pair this with a robust incident response plan: define escalation paths, automate initial containment (like isolating a compromised workload), and drill your team regularly. Speed matters—attacks unfold in minutes, and a swift response can mean the difference between a minor hiccup and a major breach.
Why It Matters Now
The importance of securing AI workloads has never been greater. AI is integral to modern businesses, powering everything from customer service chatbots to predictive analytics. However, the rapid growth of AI—seeing a 500% increase in available packages in just one year—often surpasses many organizations’ ability to protect it effectively. As a result, attackers are taking advantage of this situation, targeting AI for data theft, sabotage, or even extortion.
Take generative AI as an example: jailbreak prompts can bypass safeguards, exposing sensitive outputs. These aren’t hypothetical risks—**Sysdig’s threat research team them in the wild. Without action, your AI-driven edge could become your biggest vulnerability.
Building a Secure AI Future
Securing AI workloads involves more than just avoiding threats; it’s about building trust. Businesses can truly thrive when they can innovate confidently, knowing their AI systems are resilient. The following five steps—visibility, vulnerability management, data protection, runtime hardening, and real-time monitoring—serve as a blueprint for achieving success.
Begin with small steps: assess your AI footprint, address any critical vulnerabilities, or secure your data flows. Each action enhances your security posture. As AI continues to grow rapidly, those who prioritize security today will be better positioned for success tomorrow, protecting both their operations and their reputation.
Citations:
*https://sysdig.com/blog/5-steps-to-securing-ai-workloads/
**https://sysdig.com/blog/sysdigs-ai-workload-security-the-risks-of-rapid-ai-adoption/